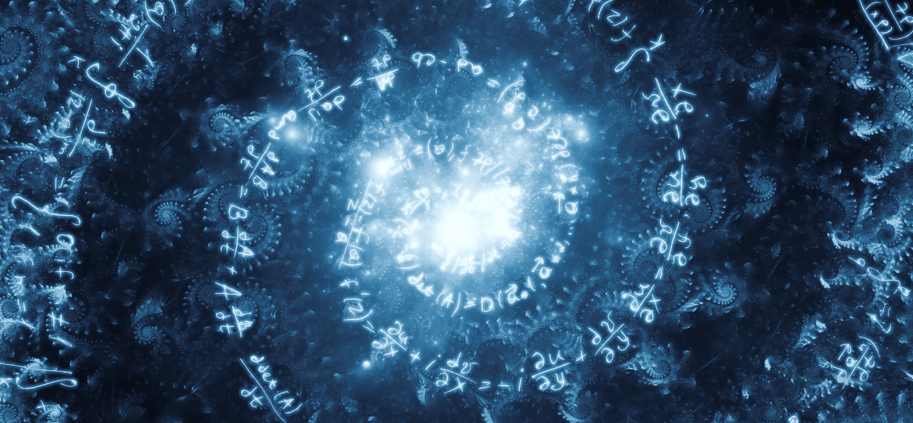
There’s a new economic force at work in the machine learning revolution that is capable of generating increasing returns to scale, much as network effects did in the internet revolution.
This force is automated learning, and its business impact comes in the form of learning effects: the more a product learns, the more valuable it becomes.
Learning effects have the potential to generate enormous economic value, as network effects do, if companies are able to close this loop and make it self-reinforcing: that is, if their products learn more because they have become more valuable.
This happens when more valuable products attract more users or customers, who provide more and richer data of the kind that enables machine learning models to make these products more valuable still, which attracts more users or customers still, and so on, creating a self-perpetuating cycle.
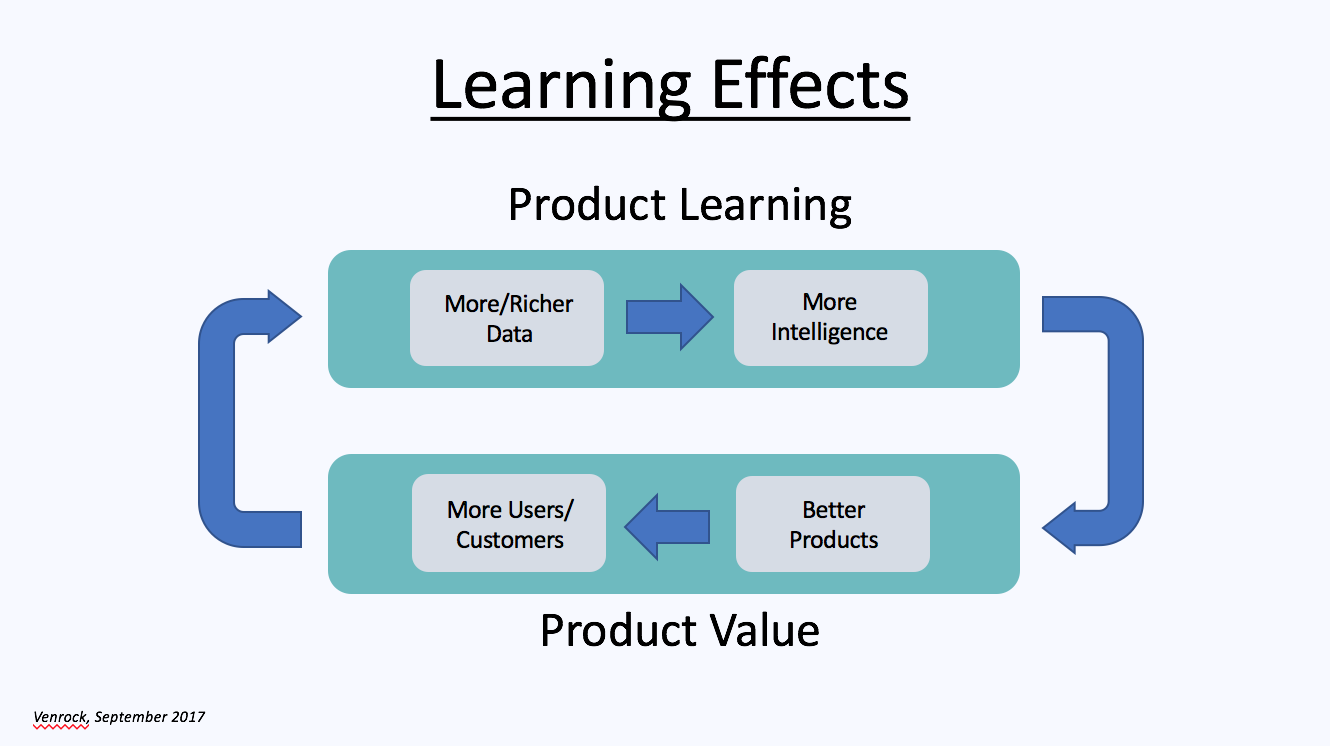
Just as network effects determined who the biggest winners of the internet revolution were, learning effects will determine who the biggest winners of the machine learning revolution will be.
Because they enable increasing returns to scale, they will similarly give rise to a set of companies that become runaway leaders – that are capable of pulling away from their competitors and continuing to increase their leads over time.